Decoding Brain Network Complexity: General Concepts and Opportunities
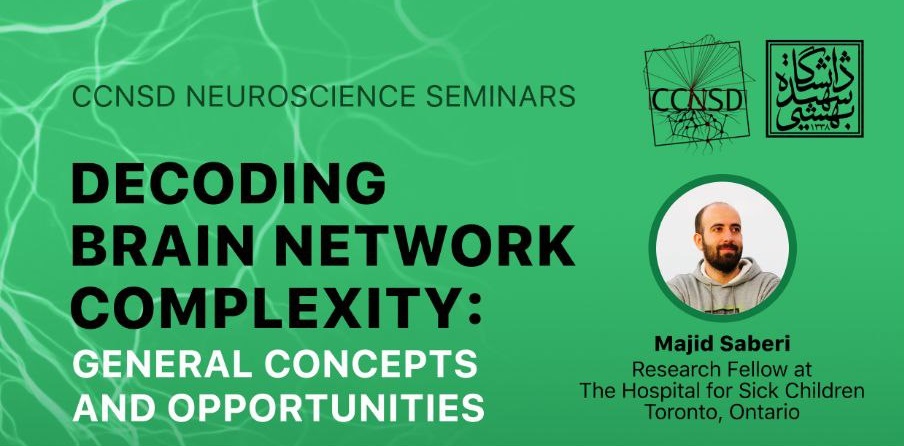
Impact of physicality on network structure
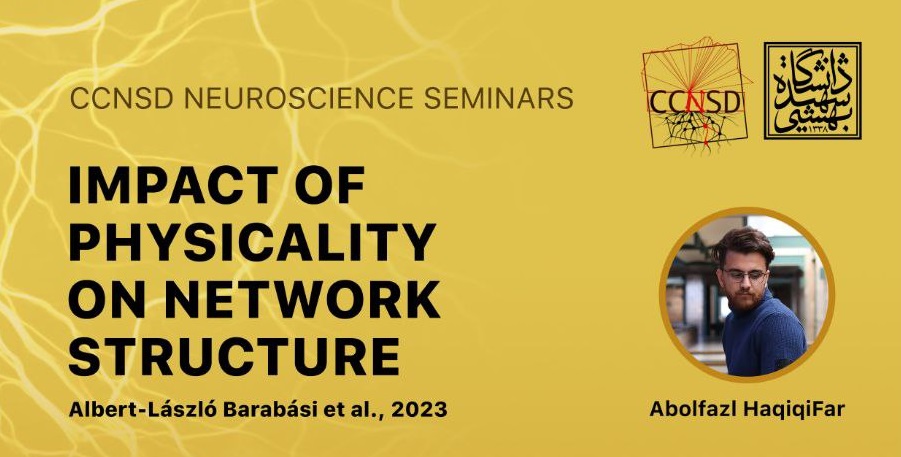
The emergence of detailed maps of physical networks, such as the brain connectome, vascular networks or composite networks in metamaterials, whose nodes and links are physical entities, has demonstrated the limits of the current network science toolset. Link physicality imposes a non-crossing condition that affects both the evolution and the structure of a network, in a way that the adjacency matrix alone—the starting point of all graph-based approaches—cannot capture. Here, we introduce a meta-graph that helps us to discover an exact mapping between linear physical networks and independent sets, which is a central concept in graph theory. The mapping allows us to analytically derive both the onset of physical effects and the emergence of a jamming transition, and to show that physicality affects the network structure even when the total volume of the links is negligible. Finally, we construct the meta-graphs of several real physical networks, which allows us to predict functional features, such as synapse formation in the brain connectome, that agree with empirical data. Overall, our results show that, to understand the evolution and behaviour of real complex networks, the role of physicality must be fully quantified.…
Is our brain chaotic?
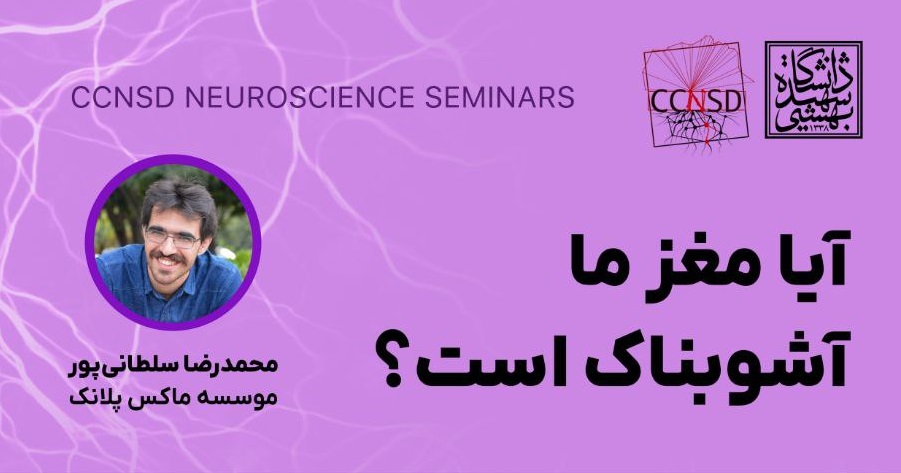